How can AI help optometrists detect more than just diabetic retinopathy? In this episode, we speak with Dr. Hadi Chakor and Yves-Stéphane Couture, the visionary minds behind DIAGNOS. Together, they share their groundbreaking partnership with IRIS, a consortium of optometry practices spanning Canada. They shared how their collaboration harnesses a vast database of patient images to redefine the approach to retinal healthcare in the Great White North. By May of 2023, IRIS and DIAGNOS had screened over 13,000 patients and developed an algorithm to help detect diabetic retinopathy, hypertensive retinopathy, and macular degeneration. Dr. Chakor and Yves also navigate through the ethical considerations of patient consent and the profound implications of AI on early retinal disease detection and microcirculation changes. Throughout the episode, they emphasize that AI isn’t here to replace us; it’s here to empower us. And that Optometrists, armed with AI, can deliver precision, compassion, and superior patient care. Join us in learning the boundless potential AI brings to the forefront of healthcare innovation.
—
Watch the episode here
Listen to the podcast here
Utilizing AI To Diagnose Retinal Disease – Dr. Hadi Chakor And Yves-Stéphane Couture, DIAGNOS
I have two amazing guests here. This is going to be one of those episodes that is going to change the way that we see the future of eyecare and, hopefully, down the road the way we practice as well. We are going to be talking about AI and the medical applications of AI in eyecare. That’s happening now, not about to happen. We’re going to be talking to the two members of the team from the company Diagnos, a developing technology that uses AI and machine learning to extract information from the retina specifically to elevate eyecare for ECPs.
I have Yves-Stéphane Couture, who has been working in tech for many years. He started in telecom, moved over to software, and now working with AI at Diagnos. He’s the VP of Sales there. I also have Dr. Hadi Chakor who is the Chief Medical Officer. He’s a medical doctor. He has a Master’s in Biomedical Engineering from the University of Montreal and has a PhD in Biomedical Engineering or what he says Preventative Medicine. He is working on creating predictive medical models, which I love that term, with Diagnos. It’s going to be exciting to talk about the company Diagnos, the technology there, and the applications of AI at large within eyecare. Thank you so much for joining me, both of you. I appreciate it.
Thank you.
Why don’t we start with a little bit of introduction, if you don’t mind, each of you take a turn. Tell me a little bit about yourself and then let’s dive into Diagnos.
My name is Hadi Chakor. I’m the Chief Medical Officer of Diagnos. I am a Medical Doctor or MD with a specialization in Ophthalmology. I did a Master’s in Biomedical Engineering. I found Diagnos in 2017. I like this company. It is the way of the future. I facilitated this predictive medicine.
That’s a very big difference. We’ve seen people who live longer, but those last years can be not great years. Living healthier is a much better option.
I’m Yves-Stéphane Couture. I’m the VP of Sales here at Diagnos. I have been in technology for many years, working in telecom, first selling to large telcos. We have Telus and all those big accounts doing fiber optics. We deployed the internet into the home, the smartphone, and everything. I saw all those changes. We brought this technology into this company to bring new services to the end users.
When I joined Diagnos in 2017, we got this technology, AI, machine learning, and computers working for us and make them work for us to enhance the quality of life of the patients, help them when they’re sick to do early detection as well as help the doctor to see more patient or help the optometrist to have more precise information so they can make a better decision. It’s all about that. It’s creating value for everybody and making sure we’re all better because we’re using technology. That’s what I like to do.
I don’t know if this is a company and a technology that too many people have heard of yet, but I’m sure it’s something that’s going to become something that we’re more familiar with over the coming years. Why don’t we talk about how it started? Let’s start a little bit with the origins of it and what it does.
I can give you a brief summary of that. I wasn’t there. Diagnos started doing all innovation and AI before it was AI. They did a few applications. The one is they work very well in the mining industry. What they did is they looked at the geophysics, collected all the data, did data mining, and did a predictive model. If you have these types of minerals and physics, then you can predict you have a mineral. When you go back and do those models over and over again and more precise in mining, you find minerals. This is very successful. They spun off this company, and now it’s living on itself. They had a couple of other technologies that they tried. It was not as successful, and it’s okay.
After that, you learn and get better. In 2009 or 2010, they moved into healthcare, starting to have a vision of what they want to do in healthcare. They’ve been there since then. They’ve been working to get some technology in there. At first, they were detecting the presence of bright and dark lesions in the retina. After that, they moved forward and I joined in 2017. This is where we took another avenue using deep learning as well. They were using machine learning to try to predict more than this presence. They are trying to understand what is it and which level of severity is deceptive.
Thank you for the backstory there. What you said right at the end is maybe an important distinction to make for us who are less knowledgeable on the AI technology type of conversation. Machine learning versus deep learning versus AI, is there a very quick distinction that you can make for us so we understand the difference?
Machine learning is when you analyze an image and the features of this image. You are trying to see what is in the image. A deep learning is a little bit more complex than that. You have a large data. As an example, diabetic retinopathy is what users seek most. There are four levels of diabetic retinopathy there, 1, 2, 3, or 4. It’s mild, moderate, severe, and proliferative. What deep learning does is you let the machine decide. You put images, the level of severity, and bring the algorithm to predict at which level of severity the image is.
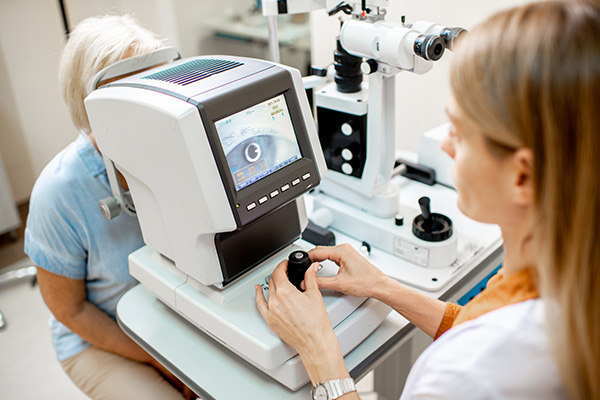
You have multiple layers to do that. You let the machine decide which characteristic in the image is going to be relevant for the algorithm to decide if it’s 1, 2, 3, or 4. You teach them 1, 2, or 3 times multiple layers on that. You take one image. It goes down in predicting which level of retinopathy it is and other levels of severity of illnesses. That’s a very high level. You predict that. You would say like, “What is the contour and color? Is it sharp?” That’s the feature base. The other one would be predicted by the level.
That makes sense in the process when I’m looking at a patient’s eye. When I’m looking at it and I’m making my notes, usually, I will describe what I’m seeing the size, location, and color, then later I have to make a diagnosis. I’m giving my assessment plan, “Here’s the diagnosis based on the feature of those things that I saw.” Machine learning is more like seeing it and describing what it looks like, and then deep learning is more like giving a definition and a diagnosis to it.
It is the cognitive process to do that using a computer.
I want to add something also because I remember when I started with machine learning and all these engineers working with diagnostics. They used to come to my office and I showed them where the disease is, and the different vision in the retina. Deep learning is another story because one of the challenges of deep learning is the need for updated data. This is the most challenging thing in deep learning, but we cannot change there because I remember the analysis of one image by using machine learning to spot them sometimes in 60 seconds or more than 1 minute. While using deep learning, it’s three seconds. The comparison between 1 image to the other is 27,000 features between two images in 2 or 3 seconds. For that, you need only updated data, but this is the challenge. You start data cleaning and validation. It’s expensive and inclusive because they can each disease own that.
There’s a lot to it, obviously. Dr. Chakor is giving a little bit more in depth about what is a difference there and why deep learning is much more detailed. Twenty-seven thousand different features in a matter of a couple of seconds is incredible. Tell us what does Diagnos do? What does it look like? A patient puts their head in it, takes a picture of the retina, and then does a bunch of analysis. Is that ultimately, on the basis, of what it does?
What we do is we use the fundus pictures and the OCT from various manufacturers in a marketplace. We analyze and detect the fundus pictures and the OCT. After that, we’ll detect analysis and rate them by the little severity and provide the response back to the healthcare professional so they can make a decision after.
The images are gathered by existing technology, whatever retinal scan or OCT that the clinic may already have. You integrate your technology with that.
We take these images and send them to our data center.
This is happening live time. As I was seeing a patient, their pictures were taken in the pretesting room before the patient sat in front of me. Those pictures have already been sent and they’ve come to my computer in the exam room with the analysis.
You can go and look at a response and make your own validation. I’m talking about you have the patients in front of you. You know it’s a story if it works with the medication the patient is taking. I can tell you a lot more. You’ve been talking to the patient about maybe the first, second, or third visit. Using all this information, you make your own diagnostic. It’s an AI system.
One thing we’re going to be talking about is whether Diagnos had a partnership or a relationship with IRIS, the group of optometry practices across Canada. I’d like to learn a bit more about it because that was a big part over the last few years, gathering a lot of data and having that normative database now to create that analysis for each of these images. Let’s talk a little bit about that. When did that partnership begin and what’s happened to cap that off?
To give you a little bit more background on that, Diagnos started research for diabetic retinopathy. The pharmaceutical company hired us to go around the globe to do a screening clinic in the States, Canada, Europe, the Middle East, and India. We acquired this very large database of 400,000 patient images. We have this huge database. We did a lot of that for pharmaceutical companies. They asked in the past.
When I joined, we started to work at the University of Montreal Teaching Hospital with CHUM. We installed our application in the endocrinology department. We can do a test for diabetic retinopathy or with diabetes and OCT endocrinologist. It is the health of the retina of my patient for diabetic retinopathy. We did this test. We worked with the ophthalmology department there. Our help was to put out the patients with severe cases with more priorities so they could be seen by an ophthalmologist. For people with a less severe case of diabetes, at least the doctor can know about it, adjust medication, and give some feedback to the patient. It was doing great there and working well.
I decided to try to go back outside and the optometrist. I met with IRIS. This is great. Retinopathy is very depressing, but this is where a broad population is. That’s important. It was not enough. This is when I’m working with Dr. Chakor. He’s been working for a long time to understand microcirculation and trying to detect cardiovascular diseases. You are looking at the microcirculation. That caught the attention of IRIS, and this is, “Now we’re talking about something that could be more interesting because I can screen my patient for illnesses related to diabetic retinopathy and maybe some of the diseases hypertensive retinopathy. Now I can propose a service what the population is and what age metric for diabetes is.”
One of the thoughts that was coming through my mind was, “Analyzing diabetic retinopathy is fine, but how much does it change my practice?” If you’re looking at the microcirculation looking for multiple different diseases, now we’re talking about what can change how I’m treating this patient in front of me significantly. Also, going back to the fact that you have deployed this technology across the world in all these different countries and populations gives you a very broad database with a lot of different patient data globally from different backgrounds. That’s huge.
We know some of the older normative databases for OCTs, and things were very narrow. When you have somebody who’s a South Asian patient coming in, all of a sudden, the database is saying they have glaucoma because it’s based on other data points that are not relevant to that background. It’s cool that you have that. Four hundred thousand patients sound like a lot of people.
Also, different populations.
That’s probably even more valuable. I skipped over all of that very important information of getting this global population, this big database, all these data points. The fact that you’ve had it deployed in a highly well-renowned hospital at the University of Montreal, you’ve had all of that, and now you have a partnership with IRIS. Tell me about that.
When we started talking with IRIS, now that we can bring this new application and get all the expertise of Dr. Chakor with retinopathy, we put a plan together or proposal to get screening data based on the specificity of the Canadian population. I proposed to them data population and use as well the annotation validation and all the data points from local clinician optometrists so we can understand what’s important to them, we can learn from them by looking at those pictures and training the algorithm that we had using verifications and more specific challenge that presents itself in Canada into the healthcare.
This is what we did. We built that together. We went to get some from the government. We were able to deploy the platform to the setting of an optometry clinic, different than the setting of a doctor. They look at different things. We had some adjustments in our software and our application to meet that. We deployed it into the first 10 clinics after that 13. We were able to gather information from 14,000 patients and multiple pieces.
It’s 14,000 patients and now you have that annotations from those doctors to give the deep learning that information to understand those conditions and those images. Dr. Chakor, anything to add to that?
That would be interesting for Dr. Chakor to talk more about retinopathy to tell us the big difference in that.
When you touch on that, the starting point is the most damage of the sugar is more on the microcirculation. The acid and resistance called is microcirculation. That’s why a patient with a diabetic condition starts to have diabetic retinopathy because of the damage of sugar directly on the microcirculation. If the patient has concerns even one step before the diabetes convention start to have this microcirculation because of the inflammation.
It’s all connected. All these patients with this condition, it’s a chronic disease. Most of the time, we have high blood pressure diagnosed by the family doctor or primary care. The damage is death on the microcirculation because of the blood pressure and also the sugar. Microcirculation, by using this type of technology, helps us to manage and also to prevent palpitation.
The sugar and high blood pressure both have an effect on microcirculation. This technology diagnosis is going to help pick up those changes in the microcirculation before there are macro changes. I thought I would clarify a little bit on that to clarify what you were saying that the damage from sugar, the elevated sugar in diabetes, and also the damage due to hypertension, is on the microcirculation. The diagnosed technology with AI and deep learning is helping us detect changes in microcirculation, which means we’ll be able to pick up changes much earlier.
In the early stage of this damage is on the retinal arteries and veins by definition which is called the artery ratio, which is the ratio between arteries and starts to be lower. We have the presence of other anomalies, but all these features are now under the radar of artificial intelligence. This is wonderful. It’s an objective user not like before. Before, it was ophthalmologists, but still, it’s subjective when you see the graph using the human eye. It’s not artificial but gives you the right view.
We assess those features of the vasculature. We look at the tortuosity and ratio, but we have biases and we’re estimating, “It changed a little bit like this,” but when you’re using a machine, you’re using technology soon going to be much more accurate and there’s no bias or at least there shouldn’t be. That’s something we could talk about a little more in a minute. It sounds like this is something that can be extremely valuable. What’s the expectation now moving forward for a few years here used within a set group of clinics within IRIS and now those tests are done and it’s assumed or concluded that it works well? What’s happening next?
It’s collecting data. It’s one thing, we did collect the data. We retrain the algorithm three times. We shared those data with IRIS and with the clinical committee as well. We made sure that what we were doing was monitoring and misunderstood and we all agreed on that. One aspect we haven’t talked about is we made sure that every patient who came in had the consent form and consented to use the data.
One thing that’s very important in AI is you need to be adequate. You need to make sure that people are well-informed of what we do because we’ve been only going to use that to retrain, as well as when we retrain, we share the information and it’s reviewed by them. That’s independent of us. We went there. We’ve done that. With IRIS, now we deploy. We’re in a phase to deploy this technology into their clinics. We have about twenty done in Quebec where we’re doing more and we’re going to start deploying that in Ontario as well.
One thing that’s very important to AI is you need to make sure that people are well-informed of what they do.
The ethical side of the conversation is something I want to talk a little bit more about. I know that’s something that’s important for you as well. You talk about having patients sign these consent forms for everything because their data technically is being used. What other types of ethical considerations do you have to consider when deploying something like this?
For us, because we’re using only a picture of the retina by using a graph or a CT, we need approval from the patient. We’re not using special data from the patient. It’s simpler. The patient gave us the consent and signed the consent form. We model that using artificial intelligence in another medical field, but we model another specialty in another scope.
One thing that we did is make sure that all the ages represented in our dataset are in IRIS and all regions as well. We want to have like a clientele that is more down Montreal only. We went to the region as well and we made sure to work very closely with the eyecare professional. In the process, we explained what we were looking out, how algorithms were working, and how we used tech. We spend a lot of time updating on how we do the classification, making sure we’re all working from the same level field as well as looking at the data and results, and making sure that they agree with it and the way the platform was making sense for them making using the right word and everything. It was a whole process and we spent a lot of time with them to make sure it was done to the highest level.
With generative AI especially, there’s a lot of talk about bias. The data output is very much dependent on the input of data. Is that something that’s a concern here as well? You’ve gone through this. You’ve collected lots of patient data. You’ve had doctors put their annotations. Is there any concern that maybe the inputs from the doctors where there is some bias integrated that might affect output?
Yes, because data training and patients are very important in AI. When you’re doing deep learning and your data is well-validated, your algorithm works better. If the data is not well-validated, we’ll have a lot of mistakes.
You’ve spent a lot of time making sure it’s valid.
We are doing a lot of tests also to make sure everything is right.
Our solution is supervised training. We don’t just upload the data, put it there, retrain, and that’s it. Everything has been looked at. It’s cleaned up and rearranged and making sure everything’s okay. If we have some doubts, we put them aside. We go verify why we have doubts. We decide if we put it in or not. That’s supervised. We have training and some new tools for this process, mini steps, 1 or 3 times before we are good to go. This is how you get very high quality and high performance.
Maybe the elephant in the room in all of this conversation is anytime AI is brought up now is, “Is this going to take my job?” Is this going to somehow exclude in the foreseeable future the optometrist from this entire equation where you stick your head in a scanner and green is good, red is bad, and see you later?
No. I can say the very opposite. An optometrist is a healthcare professional. The machine looks at the pictures and draws some classifications and deductions. The machine doesn’t explain that to the patient who doesn’t understand the history of the patient so they couldn’t put that into context. It doesn’t give advice and it’s not there to reassure the patient on what’s going on and give them the best direction, advice, and recommendation on what to do.
Looking at pictures, you can see a lot of stuff. Sometimes you think it’s one illness. You need someone else to make sure that what the machine thinks is one thing and not something else. Only a healthcare professional can decide that. It’s going to bring the optometrist more at the forefront of the first line of service for patients with eye diseases. On the opposite, it’s going to be more work for optometrists because they provide healthcare. The machine is looking for detection and all this very hard, repetitive work. The person is done by healthcare service, and this is very important because you need to put it back to the patient where the healthcare service is the center of this. That’s my take.
That puts optometrists in the front line of pre-medicare by using artificial intelligence. Take this example of high blood pressure. This is another tool for them to manage and monitor people with blood pressure conditions. That means if the microcirculation thickens, we can see easily by using artificial intelligence. In my opinion, artificial intelligence is another tool to manage and screen people.
It’s a great tool. There’s no doubt. imagine it’s going to be very helpful for practitioners who embrace it and apply it, but ultimately at least trying to quell some of those fears that some may have about technology overtaking our job or our profession. The question I wanted to ask you is should it be fear or excitement about this technology?
For me, excitement because I am MD but I was always distracted by our daily life and work. It is very difficult to have a predictive medicine, but by using artificial intelligence, that’s been facilitated. Artificial intelligence was to reshape predictive medicine and the medical practice. For me, it is not taking our job by using this. It also happened years ago about the internet and network. People are saying that we will have less job, but we have more jobs. It is the same with artificial intelligence. It is another revolution.
Artificial Intelligence is not taking our job. We do a better job by using this.
They should be very excited and they should be critical about it. There are files that they can doublecheck, but at the same time, you should embrace them because it makes the technology work for them and for their patients. Let the technology work the hard work, the tedious, repetitive tasks. AI can do it better than they can, but let’s go back and spend more time with our patients explaining what they have. Give them advice and be into caring about the relations to healthcare relationship. This is where humans are. This is where we bring value. We should let the machine do the work that the machine does better and focus on care. You should be very excited. Knowledge is available at our fingertips.
It’s very exciting. For us as eyecare providers, it’s important to not be complacent and not sit back and to actively engage and embrace the technology so we can bring it in and improve the way we take care of our patients and also not fall behind. The saying these days seems to be, “AI is not going to take our job, but someone who uses AI is going to take your job.” If we don’t embrace it, we tend to fall behind. I look forward to seeing this technology coming out more. Thank you. I appreciate that. Any final words before we wrap up?
If I have something to say, for me, the future is bright. When I started to talk about predictive medicine, I was very excited by artificial intelligence in the field. Not only longer, but healthier.
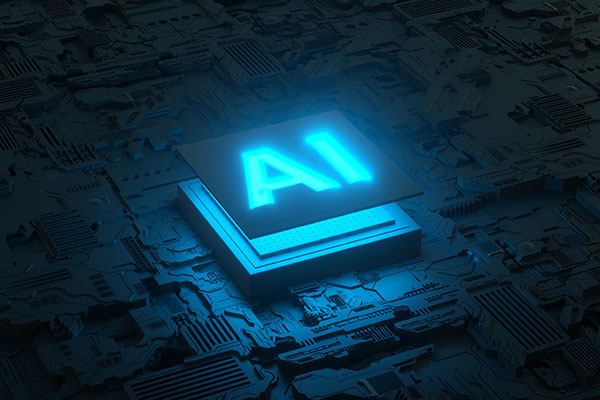
I appreciate that.
Thank you.
Before we wrap up, where can people learn more? What’s a place that someone could go to learn more about Diagnos and what’s happening there?
They can go to our website, Diagnos.com. Hopefully, we can do more interviews so we can bring more talk.
I love this. This is great. It’s something that I’m very excited about, and I know many of my colleagues are eager to learn more about what’s out there. It’s nice to be able to talk about something that’s out there implemented in practices now versus like, “This is an exciting thing coming down the road.” There’s technology being deployed that we can learn about and hopefully integrate into our practices in the near future. Thank you very much both of you for taking the time to join me. I appreciate it very much. Thank you, readers. I will see you guys in the next episode. Take care.
Important Links
About Dr. Hadi Chakor
Hadi Chakor have completed MD degree with specialisation in ophthalmology, Msc in biomedical engineering, Certified Visual Electrophysiology Specialist (ISCEV certification). During my doctoral studies (PhD in biomedical) I filled patent on screening the risk of cardio-vascular events by analysing retinal mico-vascularisation. Stronger by more than 20 years of experience and expertise in clinical and medical research field in ophthalmology and medical research.
Dr. Chakor is a chief medical officer (CMO) of global medical affairs at DIAGNOS. Inc. He is responsible of the validation of the different screening application based on artificial intelligence (AI). He is also specialist in ophthalmic visual electrodiagnostic and retinal function, he is member of the International Society for Clinical Electrophysiology of Vision (ISCEV), ARVO association for research in vision and ophthalmology and ICO (international council of ophthalmology).
- He is author of many protocols for optimization of preventive therapies, modern screening should be based on primary AI-based automatic screening tests to assess the ocular fundus. These investigations should look beyond the central macula to provide objective screening and to detect a subtle change on the retinal membrane.
- He is also an author of a new protocol Investigating the association between the prevalence of cardiovascular disease and descriptors of the retinal microvasculature in a high and normal risk population for better follow up and management of patients with systemic disease.
- He has authored and co-authored more than 50 articles and abstracts.
About Yves-Stéphane Couture
A telecommunications and technology business executive with over thirty years experience in various roles in the ICT, Healthcare industries and AI. A passionate person believing that innovation can unlock significant business value while improving health, quality of life and social well being. Talented communication leader to catalyst change in various environments.
Results oriented and success driven combined with a proven track record for applying focused skills and experience in leadership, sales, marketing and finance to increase business results for companies within a backdrop of rapid and accelerating change. A strong belief that innovation will be the key to solving the most important challenges we face in the modern world.
For the past four years, Yves-Stéphane was the leader in DIAGNOS corporate shift toward AI in optometry. Under his direction, the IRIS project was developed in collaboration with key innovators in the company. He is committed to offering AI solutions with a clear benefit for patients and the needs of healthcare professionals. Communication and multidisciplinary team management is one of his strengths to have an efficient AI at the service of patients.
Leader in business development with more than 25 years of experience in telecommunications and information technology. With his experience in team management with technology leaders such as Teleglobe, Alcatel-Lucent and Nokia, Mr. Couture has acquired several experiences with a wide variety of high-tech solutions with high added value.